Best Practices for Design and Implementation
April 23, 2024
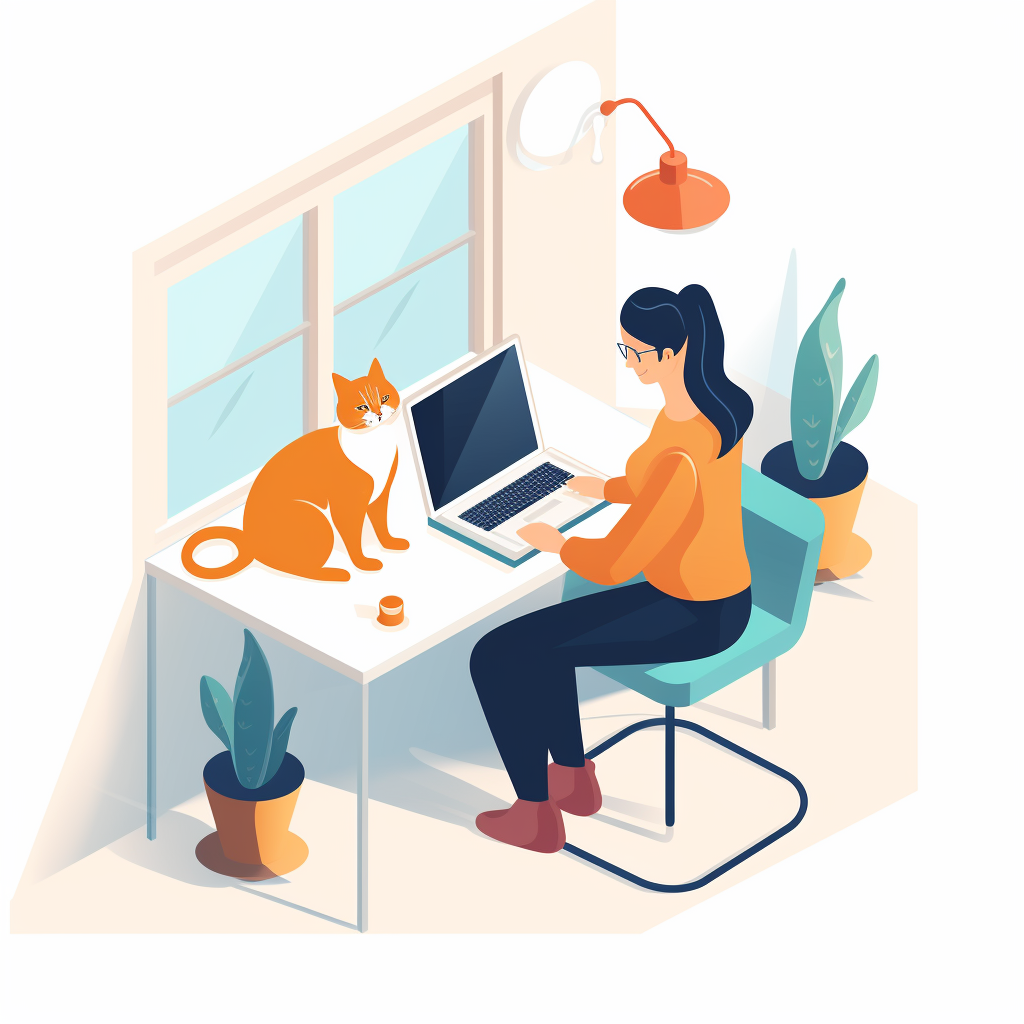
If you are designing a survey study, chances are that you are interested in the true thoughts of real people. Bots and other malicious actors need not apply; but what do we do about regular people who might not be the most attentive?
Attention, please!
Any survey study faces the challenge of grabbing and maintaining participants’ attention. When a survey is online and unsupervised, researchers are often even more concerned. One common response to this concern is to use attention checks.
Types of attention checks: Bogus items and IMCs
Ward and Meade (2023) outline two categories of attention checks: bogus items and instructional manipulation checks. Bogus items have a commonly-agreed-upon correct answer (e.g., “a dolphin is an animal”), and answering incorrectly is considered a sign of inattentiveness. Instructional manipulation checks (IMCs) instead provide a real question, but also contain (perhaps in the middle or end of the question) instructions to ignore the rest of the question and provide a specific response or other behavior (e.g., clicking somewhere on the screen).
Gummer and colleagues (2021) also describe a third type of attention check, instructed response items (IRIs), as a special kind of IMC. An IRI contains instructions hidden within one label of a grid response item, instead of in the instructions of an item. This means that an IRI assesses whether respondents have read a specific aspect of a grid item.
What do attention checks measure?
Like every survey item, an attention check measures an underlying construct (attention) imperfectly, with error (Berinsky et al., 2014). Failing an attention check item may reflect distraction, low motivation, or boredom. But according to Anduiza and Galais (2016), it may also reflect satisficing, an often-reasonable cognitive strategy of stopping once an outcome seems adequate, rather than trying to reach perfection (Brown, 2004; Simon, 1947).
Importantly, failing an attention check doesn’t always mean that a participant didn’t notice it. Sometimes, they are fully aware of it, yet actively decide not to comply (e.g., Curran & Hauser, 2019; Silber et al., 2022). It is also worth mentioning that inattentive respondents can successfully pass attention checks by random chance, depending on the design of the check.
Anduiza and Galais (2016) reviewed the literature on instructional manipulation checks (IMCs) and concluded that failing to pass one is “indeed an indicator of poorer data quality.” Supporting that point, Maniaci and Rogge (2014) found that inattentive participants are less compliant with study tasks and provide lower quality self-report data. However, failure can also correlate with research-relevant measures like demographics. For example, Anduiza and Galais (2016) found that those who failed an IMC were younger, less educated, and less interested in the survey topic than those who pass.
Warning: Side effects include…
There are also potential side effects of including IMCs in your study. IMCs, with their hidden and contradictory instructions, can lead participants to think there is “more than meets the eye” in a survey, increasing systematic thinking and therefore influencing responses on complex reasoning tasks (Hauser & Schwarz, 2015).
However, fortunately for most survey researchers, IMCs seem unlikely to impact how respondents approach standard survey questions (Hauser et al., 2016). Nevertheless, repeated IMCs can have undesirable impacts on respondents, including discomfort and unease (Anduiza & Galais, 2016). These authors even suggest that researchers may be better off without IMCs at all.
Best practices for designing attention checks…
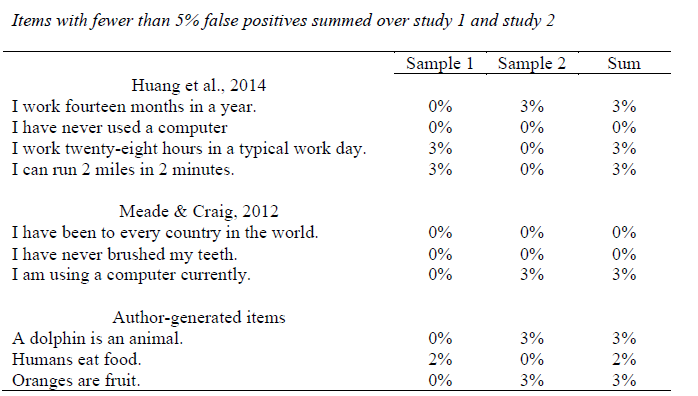
If you have decided to include attention checks in your survey, you are in good company. In a recent overarching review of inattentive responding, Ward and Meade (2023) encourage the inclusion of attention checks, particularly in “high-risk” situations, such as extremely long or repetitive surveys. So what should they look like?
First, it is best to think of attention as a spectrum (Berinsky et al., 2014). Further, social scientists mostly think about data quality in terms of an entire survey (Gummer et al., 2021). Therefore, many experts suggest including multiple attention check items spread through a survey and treating them as a scale (Curran & Hauser, 2019; Muszyński, 2023). However, if you absolutely must use an attention check to instantly screen participants out, then it’s most ethical to use questions that are brief, simple, and appear within the first few minutes of a survey, if possible (Moss et al., 2023).
In terms of the items themselves, some experts suggest using short, covert attention checks in order to reduce noncompliance, frustration, or other negative side effects (Muszyński, 2023). In general, it is better to select items by building off of previous research, rather than starting from scratch (Curran & Hauser, 2019). This is because questions can differ significantly in how well they identify inattentive participants while not wrongly catching attentive ones. Curran and Hauser (2019) recommend using a combination of the strongest items from multiple previously-tested scales, such as those in Table 3 of their paper.
You should keep in mind, however, that previous research may be less relevant if your target population differs significantly from the population in the original study (e.g., large differences in their knowledge or culture). In that case, try to conduct at least some minimal cognitive interviewing with members of your target population, to check for culturally-bounded understanding.
How should we use attention check data?
As it turns out, excluding participants based on attention check failures has unclear benefits and some clear downsides. For example, we can look at the impact on statistical power. Excluding inattentive respondents reduces noise (which increases power) but decreases sample sizes (which decreases power); the ultimate impact on power depends on which of those two influences is stronger in a specific case (see, e.g. Ward & Meade, 2023). Further, Berinsky et al. (2014) point out that lack of attention is a condition in the real world. For survey experiments, then, excluding less attentive respondents contributes to overestimating treatment effects through artificially inflating attention.
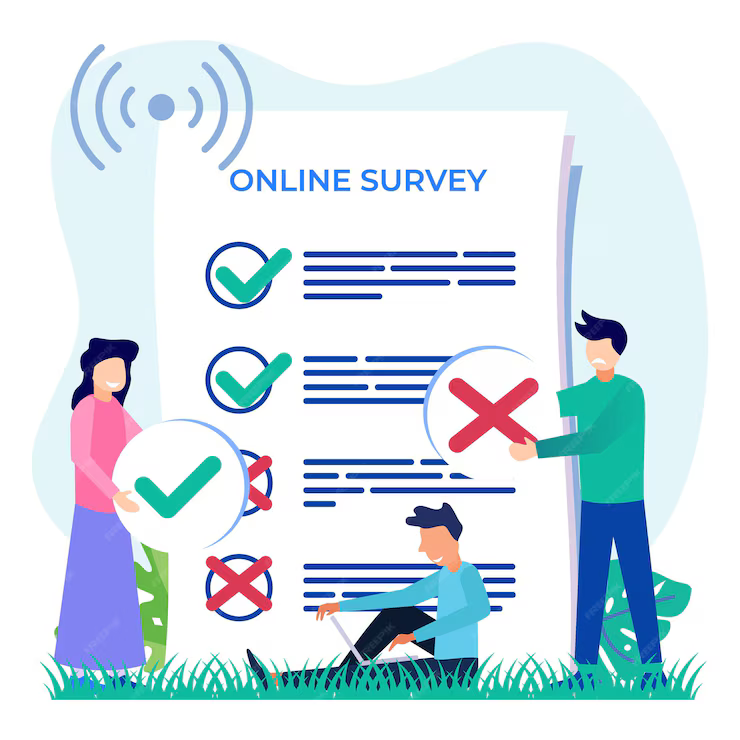
Some experts even argue against removing extremely careless respondents. Non-probabilistic online surveys typically over-represent certain categories of internet users including those who are more-educated and more interested in the topic at hand; Anduiza and Galais (2016) warn that removing respondents who fail attention screeners would mean dropping those who are more similar to the average member of the population. As a result, exclusion carries a significant risk of severely biasing estimates (Anduiza & Galais, 2016; Berinsky et al., 2014). Instead, experts including Berinksy and colleagues (2014) suggest that researchers can “balance the goals of internal and external validity by presenting results conditional on different levels of attention.” They go on to explain, “[p]resenting stratified results and considering how the culled sample affects one’s findings allows researchers to benefit from Screener questions while avoiding the drawbacks.”
Conclusion
After carefully selecting each word of your survey, it can be both demoralizing and concerning that participants would skim a question. Nevertheless, imperfectly attentive participants are often more representative of the real world, and removing their data carries severe risks for external validity. The scientific literature on attention checks suggests the following: 1) favor bogus items over IMCs; 2) attention checks are most meaningful when multi-part, but carefully consider the participant burden costs; 3) rather than excluding inattentive participants, show how attention relates to your variables of interest.
If you would like to discuss how we can help you implement your research project from end to end, book a call with me.
References
Anduiza, E., & Galais, C. (2016). Answering Without Reading: IMCs and Strong Satisficing in Online Surveys. International Journal of Public Opinion Research, 29, 497-519. https://doi.org/10.1093/ijpor/edw007
Berinsky, A. J., Margolis, M. F., & Sances, M. W. (2014). Separating the shirkers from the workers? Making sure respondents pay attention on self‐administered surveys. American journal of political science, 58(3), 739-753. https://doi.org/10.1111/ajps.12081
Brown, R. (2004). Consideration of the origin of Herbert Simon’s theory of “satisficing”(1933‐1947). Management Decision, 42(10), 1240-1256. https://doi.org/10.1108/00251740410568944
Curran, P. G., & Hauser, K. A. (2019, July 29). I’m paid biweekly, just not by leprechauns: Evaluating valid-but-incorrect response rates to attention check items. DOI: https://doi.org/10.31234/osf.io/d8hgr
Gummer, T., Roßmann, J., & Silber, H. (2021). Using instructed response items as attention checks in web surveys: Properties and implementation. Sociological Methods & Research, 50(1), 238-264. https://doi.org/10.1177/0049124118769083
Hauser, D. J., & Schwarz, N. (2015). It’s a Trap! Instructional Manipulation Checks Prompt Systematic Thinking on “Tricky” Tasks. SAGE Open, 5(2). https://doi.org/10.1177/2158244015584617
Hauser, D. J., Sunderrajan, A., Natarajan, M., & Schwarz, N. (2016). Prior exposure to instructional manipulation checks does not attenuate survey context effects driven by satisficing or gricean norms. Methods, data, analyses: a journal for quantitative methods and survey methodology (mda), 10(2), 195-220. https://doi.org/10.12758/mda.2016.008
Maniaci, M.R., & Rogge, R.D. (2014). Caring about carelessness: Participant inattention and its effects on research. Journal of Research in Personality, 48, 61-83. https://doi.org/10.1016/j.jrp.2013.09.008
Moss, A.J., Hauser, D.J., Rosenzweig C., Jaffe, S., Robinson, J., Litman, L. (2023). Using Market-Research Panels for Behavioral Science: An Overview and Tutorial. Advances in Methods and Practices in Psychological Science. 6(2). https://doi.org/10.1177/25152459221140388
Muszyński, M. (2023). Attention checks and how to use them: Review and practical recommendations. Ask Research and Methods. https://doi.org/10.18061/ask.v32i1.0001
Silber, H., Roßmann, J., & Gummer, T. (2022). The issue of noncompliance in attention check questions: False positives in instructed response items. Field Methods, 34(4), 346-360. https://doi.org/10.1177/1525822X221115830
Simon, Herbert A. (1947). Administrative Behavior: a Study of Decision-Making Processes in Administrative Organization (1st ed.). New York: Macmillan.
Ward, M. K., & Meade, A. W. (2023). Dealing with careless responding in survey data: Prevention, identification, and recommended best practices. Annual Review of Psychology, 74, 577-596. https://doi.org/10.1146/annurev-psych-040422-045007
About the Author