Classic Choice Modelling Still Beats AI at Predicting Human Decisions
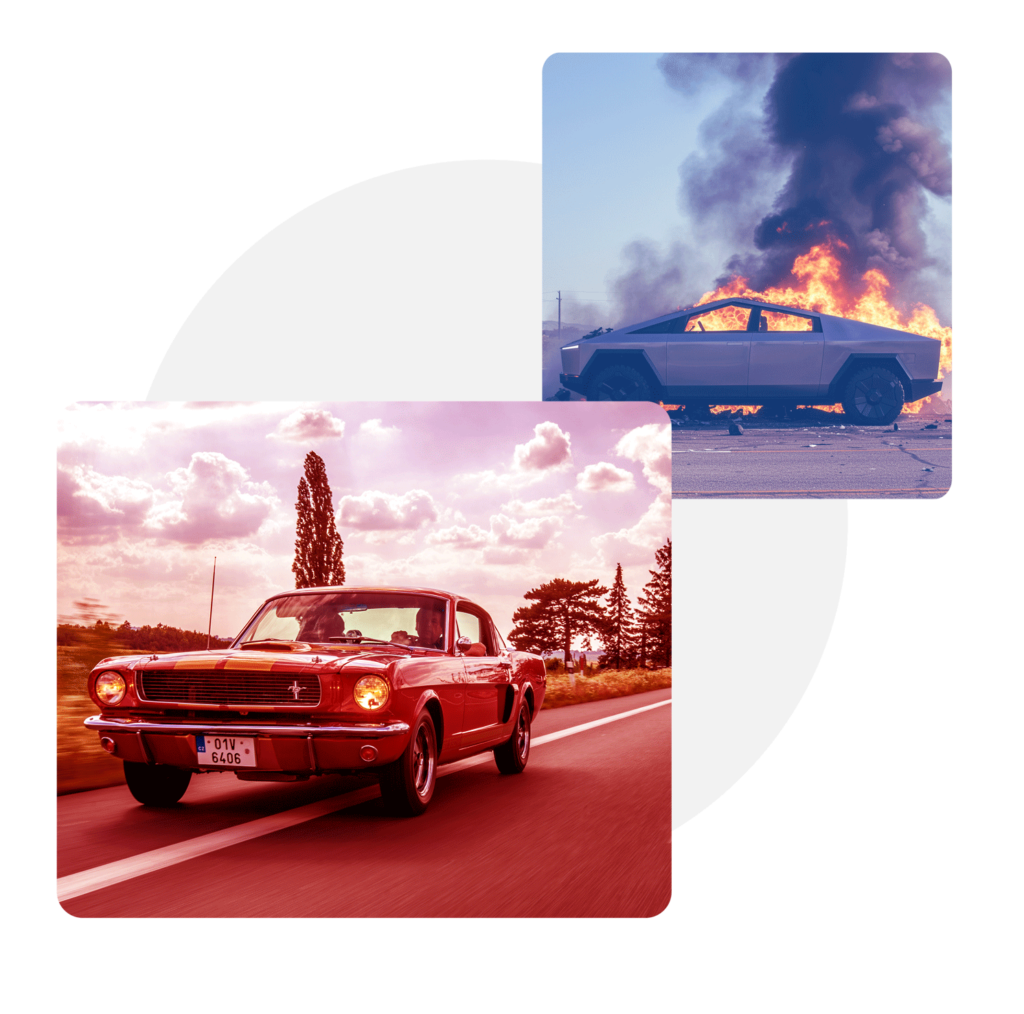
Classic Choice Models
still beat AI at Predicting Human Decisions
When it comes to predicting how people make choices, like picking a mode of transportation or choosing between products, data scientists may instinctively reach for AI or machine learning tools. But new research suggests that they might want to reconsider.
A recent study published in Transportation Research puts traditional choice modelling head-to-head with cutting-edge machine learning, and the results are surprising: choice models consistently outperform machine learning in predictive power. Despite all the hype around AI and neural networks, the good old-fashioned models based on economic theory still come out on top.
Here’s why.
1. Choice Modelling Predicts Better
The study evaluated 945 models across 22 datasets and found that discrete choice models—like multinomial logit and nested logit—often predicted human choices more accurately than complex machine learning models like random forests or neural networks. That’s a big deal, especially in fields where accurate predictions drive policy or investment decisions.
2. Rooted in Economic Theory
Unlike machine learning, which focuses on patterns in the data, choice modelling is grounded in economic theory. It assumes that individuals make decisions to maximiae their utility – essentially, their satisfaction. This makes the models not just predictive, but also explanatory. You’re not just getting a “what,” you’re getting a “why.”
3. Interpretability is Built In
One of the biggest challenges with machine learning models is the “black box” problem – it’s hard to know why the model makes certain predictions. In contrast, choice models are transparent and interpretable. They offer clear insight into the influence of different variables on decision-making, which is essential for researchers, policymakers, and businesses alike.
Final Thoughts
Machine learning has its strengths, especially in processing massive amounts of unstructured data. But when it comes to modeling human decisions – especially in transportation, marketing, and economics – choice modelling isn’t just competitive, it’s often better.
The latest isn’t always the greatest. Scientists need more than black box AI mimicry in their reseach, they need tools like Choice Modelling, that understands how people actually choose.
To learn more about choice modelling, why not join the course in Mallorca this year?
References:
- Hess, S., et al. (2023). Machine learning and discrete choice models: Two sides of the same coin? Transportation Research Part A: Policy and Practice.
Full paper on ScienceDirect - Katsikopoulos, K. V., et al. (2024). Predicting destination choice with discrete choice and machine learning models. European Transport Research Review
- Kariv, S. (n.d.). Economic foundations of discrete choice. UC Berkeley Lecture Notes
- Boos, D., et al. (2024). Explainable AI in decision-making models: A critical comparison. Business & Information Systems Engineering